Why productivity growth is declining
The consequences of lower productivity growth over the past few years are palpable. For instance, it is estimated that, in 2015, the average US citizen would have earned an extra USD 8,400 if productivity had grown at the same rate between 2005 and 2015 as between 1995 and 2004.1 Moreover, as shown by the article «The technological revolution and slowdown in productivity» in this Dossier, should the current trend continue, by 2027 the average American will have earned USD 13,400 less. So what are the factors behind this slowdown? That is what we will look at here.
Slowdown? What slowdown?
The drop-off in productivity growth is particularly surprising given the huge technological advances of the past few decades.2 Since most of these advances have been related to information and communication technologies (ICTs), whose productivity is particularly difficult to measure, one of the possible explanations is that the official statistics do not reflect the real state of productivity.
Firstly, it is more complicated to measure improvements in quality and the price trend for a specific product over time than might be supposed. This is partly because products evolve continuously. For example, if we construct a price index for TV sets as from the 1950s, we must compare a black and white set (which cost 30,000 pesetas in 1956 or, adjusted for inflation, around EUR 7,000 today) with the first colour sets and today’s smart HD TVs (which can be bought for under EUR 300). This evolution from a EUR 7,000 to a EUR 300 TV set is a clear reflection of productivity gains but the extent of this improvement is not only measured in price; we must also take the improved quality into account, something that is difficult to quantify in economic terms. Given the current context of fast technological improvements related to ICTs, there are indications that official statistics underestimate price index reductions3 and quality improvements caused by digital technology and therefore underestimate productivity growth. There is also evidence that official statistics underestimate gains in productivity resulting from an increase in international trade.4
In a study for the US, Byrne and Fernald, from the US Federal Reserve, and Reinsdorf, from the International Monetary Fund (IMF), constructed price indexes that provide a more accurate picture of the trend in quality and price of IT-related products and estimate the impact on aggregate productivity. The conclusion drawn by Byrne and colleagues is that there is a mismeasurement problem and, once corrected, the estimated «level» of productivity increases significantly. However, this increase occurs more or less evenly over time, so it does not substantially affect the slowdown in productivity «growth». If adjusted for IT-related mismeasurement, they estimate that annual US labour productivity growth would increase by +0.21 pp between 1978 and 1995, by +0.38 pp between 1995 and 2004 and by +0.19 pp between 2004 and 2014.
Secondly, another factor that makes it more complicated to measure productivity is the reallocation, by multinationals, of the property of intangible capital and earnings between parent and subsidiary companies located in different jurisdictions. This effect can be significant in quantitative terms because, as shown by Guvenen et al.,5 multinationals account for over 25% of the aggregate value added of the US economy, are significant holders of intangible capital and have considerably increased their global business. To illustrate this, let us assume that an imaginary company, Big Eyeglasses Ltd., designs glasses in the US and sells them at USD 100 per pair. The company outsources production to an Asian manufacturer, at a cost of USD 80 per pair, earning a profit of USD 20 per unit. The contribution to US GDP, in terms of the value added of the design, is USD 20. But if Big Eyeglasses Ltd. assigns ownership of the design to a subsidiary located in a more tax-friendly jurisdiction, paying this subsidiary USD 15 per unit for copyright, the official statistics register the contribution to US GDP as just USD 5. Guvenen and co. reassign the earnings of multinationals to achieve a more accurate reflection of the true value added in each jurisdiction. After this adjustment, they estimate that US productivity growth increases by «just» 0.25 pp annually between 2002 and 2008. Once again, there is a problem of measurement but this does not have a quantitatively significant effect.
Finally, a third aspect of the mismeasurement theory is based on the increasing importance of free goods and services. Most of the new technologies appearing on the market since 2004 (e.g. smartphones and social media) involve the consumption of time-intensive products (implying they add value for their users) but do not impose a high monetary cost on their consumers. In fact, most of these products only generate income indirectly through advertising. National account data treat these as intermediate input in the production process (not as a final product). Consequently they are not directly included in the calculation of GDP. For instance, on a national accounts basis, a film shown openly on a TV channel one Saturday evening is treated as a production cost for those companies advertising on the same channel. Similarly, the income earned by Facebook, Google and other companies offering free goods and services financed via advertising is treated as an intermediate cost and excluded from the direct GDP calculation (since this only includes final products).6
So could the slowdown observed in productivity be merely a reflection of the difficulties in measuring economic activity brought about by new technologies? Let us go back to our example of the TV film and imagine it as a transaction: a family pays for the right to enjoy the film by accepting to see adverts. To put a value on this transaction in monetary terms, we could use the advertising income received by the TV channel, which would then be considered as final consumption by households (instead of intermediate consumption by advertisers) and would be included directly in the GDP calculation. This is the approach proposed by Nakamura and Soloveichik (2015)7 who estimate that, in this case and in aggregate terms, the «level» of US GDP would increase by 0.5%. However, this figure is fairly constant over time and therefore does not affect productivity «growth» significantly. Another option is to estimate the monetary value of leisure, since free goods are consumed during people’s leisure time. Syverson (2016) has done this and found that, under the most favourable assumptions for the mismeasurement theory,8 free online goods could explain slightly less than one third of the slowdown in productivity, a very significant figure but still not enough.9
In short, although new technologies have made it more difficult to measure economic activity, mismeasurement does not seem to lie behind the slowdown in productivity.10
Underlying factors
Some economists have argued that the slowdown in productivity is actually a return to normal.11 As shown in the first chart, between 1995 and 2004 US productivity growth speeded up temporarily, attributed to the spread of computers and the internet. If we ignore this episode, we are left with a slowdown that dates back to the 1970s, reflecting the end of the spread of ideas from the first and second industrial revolution. In fact, some of the innovations that triggered these revolutions, such as the railways, internal combustion engine and electricity, have formed the basis of new technological developments such as the growth and sophistication of the transport network, central heating and air conditioning and domestic appliances, which continued to boost economic growth until the second half of the 20th century. However, according to these economists, the slowdown observed since 1970 suggests that information technology will have more transitory impact and will not alter either productivity or our standard of living so fundamentally.
How can we reconcile this relatively pessimistic view with the many technological advances occurring in the areas of artificial intelligence and big data? One possibility is that we are going through a transition in which companies and consumers are still learning to use new technologies effectively. Ultimately, the long time taken for the effects of the first two industrial revolutions to be truly felt suggests that innovations take time to permeate the whole economy.
In fact, if we look beyond the aggregate figures, there are signs that firm-level productivity is still growing at a good pace. In an OECD study, Andrews, Criscuolo and Gal analysed the productivity trend in a large number of firms across 24 countries between 1997 and 2014.12 The authors constructed a «productivity frontier», in which only the top 5% of firms in terms of labour productivity were included, for each year and each branch of economic activity. The study analysed the trend in this frontier over time. Their findings show that weak aggregate productivity data obscure a duality between more productive companies, or «frontier firms», and the rest, or «laggards». In manufacturing, frontier firm productivity increased by 2.8% per year between 2001 and 2013 whereas growth for the laggards was 0.6%. The gap is even wider in the services sector: the productivity of frontier firms grew at an annual rate of 3.6% while for laggards it grew by just 0.4%.
What is the reason for this gap? The analysis carried out by Andrews et al. indicates that these differences do not come from capital deepening. But they do find evidence of «winner takes all» enterprises, as well as stalling technological diffusion. In other words, on the one hand frontier companies win market share, especially in ICT-related sectors while, over time, the number of firms crossing the frontier in either direction decreases. For example, in the services sector, 50% of the frontier firms between 2001 and 2003 had also formed part of 10% of the most productive firms two years previously, while between 2011 and 2013 the proportion had grown to 63%.
In short, the findings of Andrews et al. help us to reconcile the slowdown in aggregate productivity with the emergence of new technological innovations. The key is that digital innovations are intensive in intangible capital and allow products and information to be reproduced at a marginal cost which is close to zero. They therefore make it easier for such a producer to take over most of the market. This results in a two-speed economy, with a widening gap between firms with high productivity and investment in intangible capital and firms with low productivity and little investment in intangible capital.
Productivity and the macroeconomic cycle
In addition to the aforementioned underlying factors, the severe recession that most developed countries have gone through in the past few years may have also helped to slow down productivity gains.13 In general, productivity growth tends to be procyclical: it increases in expansionary periods and decreases in recessions. A priori, this behaviour may seem counterintuitive. In fact, early in the 20th century, when data was limited, productivity was thought to be countercyclical. Wesley Mitchell, for instance, assumed that, during a recession, productivity increased because less productive workers were the first to be fired.14 On the other hand, during an economic boom he supposed that productivity fell due to the scarcity of labour, forcing firms to hire less productive workers to meet demand. Although this argument appears reasonable, as can be seen in the second chart it is not supported by the data, except in the case of Spain (a discussion we will postpone for the time being).15
The reluctance of firms to fire workers in a recession is the main reason for productivity’s procyclical behaviour.16 The empirical evidence available shows that the costs of recruiting and training workers (and also of firing them) are enough to persuade many firms to retain part of their workforce during a recession even though they may not be fully utilised. As far as possible, companies try to avoid incurring the aforementioned costs and ensure they have the best human capital available for when the tide turns again.17
If, as we have noted, the reasons why productivity is procyclical in most developed countries are positive, this is not good news for Spain, where productivity is countercyclical. In fact, the relatively large share of temporary employment in Spain’s labour market lies behind this phenomenon, since it means that adjustments made bycompaniess during recessions focus mostly on the labour factor.
The impact of the financial crisis
Apart from productivity’s procyclical nature, there are also concerns that, due to its nature and severity, the effects of the crisis affecting most developed countries can still be felt today. The third chart illustrates such concerns: the average productivity growth of the largest developed economies in the current expansionary phase is lower than that observed in the last expansionary phase.
As we have already noted, some long-run factors lie behind this slowdown. However, productivity growth has also been affected by dynamics originating in the financial crisis. One key factor is the decline in investment. Public investment has been hampered by the high debt incurred by many countries. Private investment also slumped during the crisis, mostly due to the drop in demand but also because of the high uncertainty which continued throughout the crisis and into the first few years of the economic recovery. For example, compared with the period 1970-2000, between 2012 and 2016 the percentage of GDP allocated to gross fixed capital formation fell on average by 2.6 pp in the US, by 5.6 pp in the UK, 4.5 pp in Germany, 5.2 pp in Italy and 4.2 pp in Spain. The IMF estimates that this lower investment lies behind 0.2 pp of the decrease in growth in total factor productivity (TFP) during the period 2008-2014.
As already mentioned, to some extent the drop-off in investment was particularly severe because of the high uncertainty surrounding the recessionary episode and the initial phase of the recovery. There was also the financial crisis and the consequent slump in credit, which also limited the capacity of many firms to invest. A recent study by Duval, Hong and Timmer shows that those companies which found it more difficult to get credit during the crisis in the US posted larger declines in TFP.18,19 The authors also observed that, within this same group of firms, intangible capital investment was particularly hard hit. Both facts could be related, considering that intangible assets, unlike a machine or property, are unlikely to be accepted as collateral.
The future of productivity
Although it would be natural to end this article with a prediction for the future, the accuracy of previous predictions regarding technology and productivity does not encourage optimism. For example, in 1927 one of the top men at Warner Brothers wondered, rhetorically, whether anyone would ever actually want to hear the actors’ voices. Along the same lines, in 1987, the Nobel Prize-winner for Economics, Robert Solow, stated that «you can see the computer age everywhere but in the productivity statistics» (nevertheless, note the upswing between 1995 and 2004 in the first chart). Instead of making predictions, our conclusions point to the need for a clear agenda of public policies to stimulate productivity gains, an issue addressed by the Editorial in this Monthly Report.
Oriol Carreras Baquer and Adrià Morron Salmeron
Macroeconomics Unit and Financial Markets Unit, Strategic Planning and Research Department, CaixaBank
1. See Syverson, C. (2016), «Challenges to Mismeasurement Explanations for the U.S. Productivity Slowdown», NBER Working Paper.
2. For more details on these advances, see the article «Writing the future: the technological paradigm shift and the new economy» in this Dossier.
3. See Byrne, M., Fernald, J. G. and Reinsdorf, M. B. (2016), «Does the United States have a productivity slowdown or a measurement problem?», Brookings Papers on Economic Activity.
4. Specifically, they do not accurately reflect the reduction in costs occurring when a producer that buys its intermediate inputs from a domestic producer starts to buy these from a cheaper foreign producer.
5. See Guvenen, R. J. et al. (2017), «Offshore Profit Shifting and Domestic Productivity Measurement», NBER Working Paper.
6. See the article «Even free things have a price» in the Dossier «National accounts in the digital era» in MR11/2014.
7. See Nakamura, L. and Soloveichik, R. (2015), «Valuing “Free” Media Across Countries in GDP», Federal Reserve Bank of Philadelphia Working Paper.
8. Assumptions in terms of leisure time devoted to consuming digital products and the monetary value assigned to this.
9. See Byrne et al. (2016), who combine different approaches and also reveal that free digital services are not significant enough, in quantitative terms, to explain the productivity slowdown.
10. There are another two reasons against the mismeasurement hypothesis. First, the slowdown has also occurred in sectors where productivity is measured reasonably accurately, such as retail and manufacturing. Second, in a cross-country analysis, no connection has been observed between the size of the slowdown and the relative importance of ICTs in each economy.
11. See Gordon, R. (2012), «Is US economic growth over? Faltering innovation confronts six headwinds», NBER Working Paper.
12. See Andrews et al. (2016), «The global productivity slowdown, technology divergence and public policy: a firm level perspective», Hutchins Center Working Paper.
13. Unless noted otherwise, whenever we mention productivity, this will be defined as labour productivity: GDP per hour worked.
14. Mitchell collaborated in founding the National Bureau of Economic Research (NBER) and was its Director.
15. In 1913, there was very little empirical evidence available on productivity. It was not until around 1950 that reliable data were available on productivity to evaluate its cyclical behaviour.
16. See Biddle, J. E. (2014), «Retrospectives: The Cyclical Behavior of Labor Productivity and the Emergence of the Labor Hoarding Concept», Journal of Economic Perspectives, vol. 28, no. 2, p. 212.
17. The extent to which workers are retained also depends on the company’s view of how long the recession will last.
18. See Duval, R., Hong, G. H. and Timmer, Y. (2017), «Financial Frictions and the Great Productivity Slowdown», IMF Working Paper.
19. The authors classify companies by their leverage ratio and share of debt maturing within a year at the end of 2007. Those with a high leverage ratio or high financial vulnerability are classified as companies with difficulties in accessing credit. To counter concerns that the decline observed in the productivity of companies with difficulties in accessing credit could be due to them being relatively unproductive firms, the authors show that TFP growth among both types of company was similar before the financial crisis.
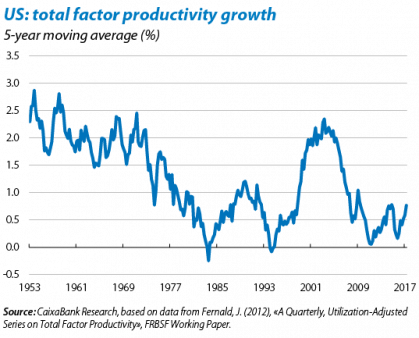
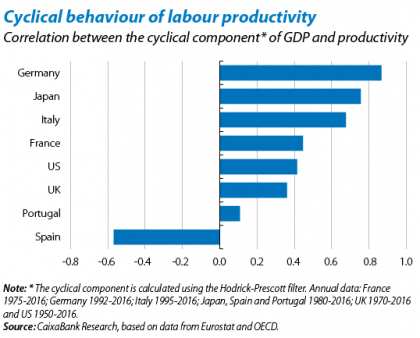
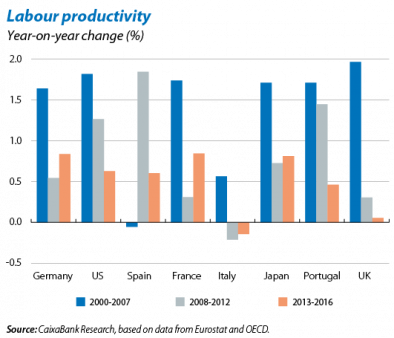